Unlocking the Potential of Labelling Tool Machine Learning for Your Business
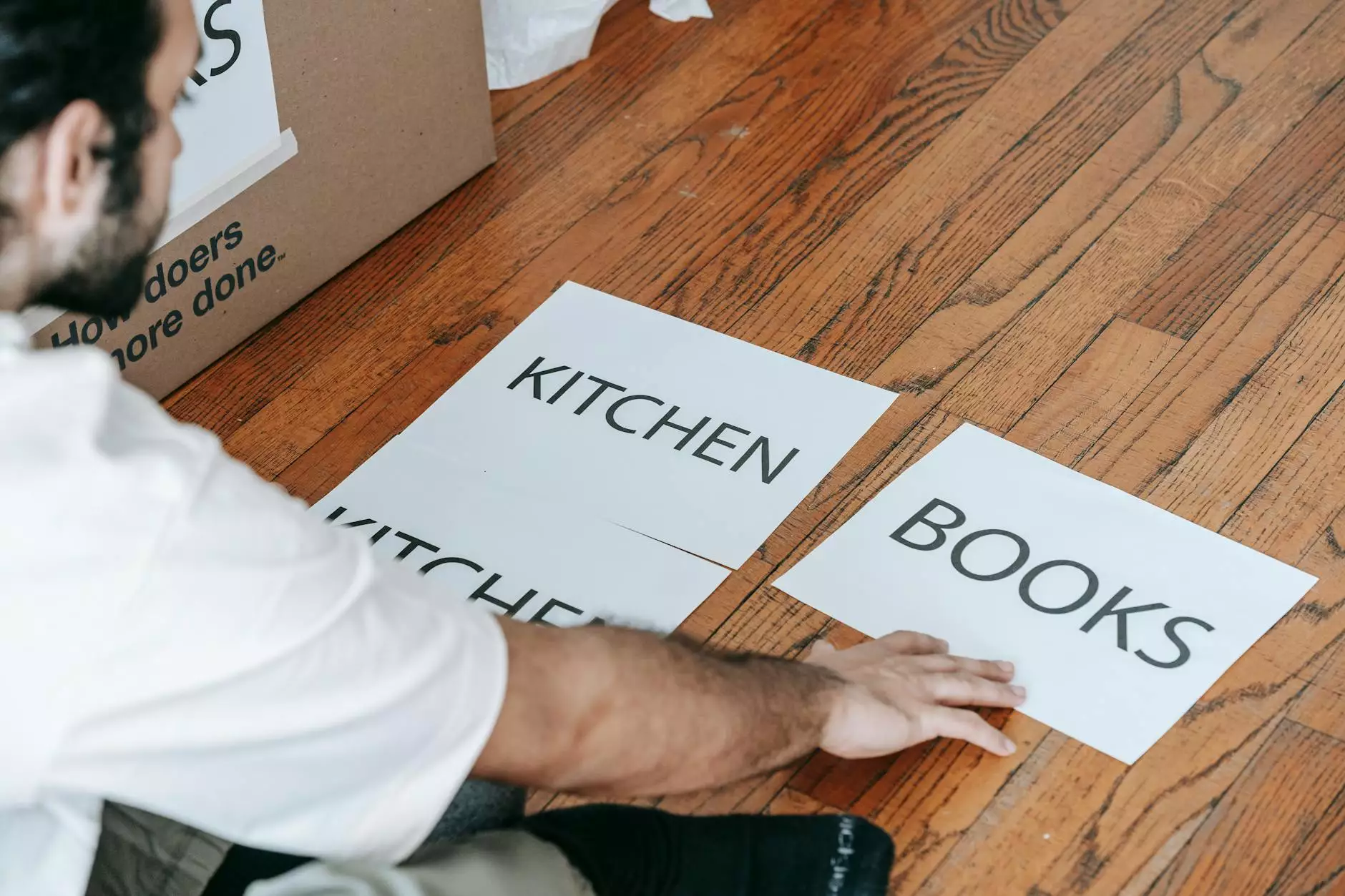
Labelling tool machine learning has transformed the way businesses manage and interpret data. In an era where the amount of data generated daily is staggering, having an efficient annotation and labelling process is crucial. This article delves deep into how these tools work, their benefits, and how businesses can leverage them to gain insights and drive decision-making.
Understanding the Basics of Machine Learning and Data Annotation
Machine learning (ML) refers to the study of algorithms and statistical models that enable computer systems to perform tasks without explicit instructions, relying on patterns and inference. At the core of machine learning is data, which needs to be accurately labelled to train models effectively.
What is Data Annotation?
Data annotation involves the process of identifying, labeling, and categorizing data. This could include anything from tagging images, transcribing audio files, or marking segments of text as relevant or irrelevant. Data annotation is essential because:
- Improves Model Accuracy: Well-annotated data leads to better model performance.
- Ensures Quality Control: High-quality annotations allow for efficient model tuning and validation.
- Facilitates Real-World Applications: Annotated data helps implement machine learning solutions in various applications including healthcare, finance, and autonomous driving.
The Role of Labelling Tools in Machine Learning
Labelling tools are software applications specifically designed to assist in the annotation process. They streamline how data is labelled and make it easier for businesses to handle large datasets.
Features of Effective Labelling Tools
While there are numerous labelling tools available, the best ones share certain features:
- User-Friendly Interface: A clean, intuitive design helps annotators work quickly and efficiently.
- Automation Capabilities: Smart automation features can speed up annotation by predicting and suggesting labels.
- Customizability: The ability to tailor the tool to specific data types and use cases.
- Integration Options: Seamless integration with existing data workflows enhances usability.
- Quality Assurance Mechanisms: Built-in checks and balances to maintain high-quality annotation standards.
Advantages of Using a Labelling Tool for Machine Learning
The use of a labelling tool can bring incredible advantages to businesses seeking to implement machine learning solutions:
1. Enhanced Efficiency
Using a labelling tool drastically reduces the time spent on data annotation. With features like batch processing and automation, teams can work on larger datasets faster than ever before.
2. Scalability
As your business grows, so does the volume of data. A robust labelling tool allows you to scale your annotation processes according to your business needs without compromising quality.
3. Improved Collaboration and Workflow
Most modern labelling tools provide collaborative features that allow multiple annotators to work simultaneously. Teams can communicate directly through the tool, allowing for complete transparency and improved workflow.
4. Cost-Effectiveness
While investing in a labelling tool may seem daunting, the long-term savings through increased productivity and reduced error rates make it a worthwhile investment. Effective annotation leads to faster project completions and better models, ultimately contributing to the bottom line.
Choosing the Right Labelling Tool for Your Needs
Selecting the appropriate labelling tool is essential for optimizing your machine learning projects. Here are some considerations to keep in mind:
1. Identify Your Specific Use Case
Different projects may require different annotation types—image segmentation, text annotation, or audio transcription. Understanding your unique needs will help you zero in on the right tool.
2. Assess Your Team's Skill Level
Make sure to consider the technical proficiency of your team. A user-friendly tool can mitigate the learning curve and ease the transition to new workflows.
3. Explore Available Integrations
Your labelling tool should seamlessly integrate into your existing data ecosystem. This will ensure that data flow is uninterrupted and that you can operate without needing to overhaul your current systems.
4. Evaluate Support and Resources
Support options, documentation, and training resources are vital in helping your team maximize the use of the tool.
Examples of Popular Labelling Tools in Machine Learning
There are several well-known labelling tools that businesses can consider:
1. Labelbox
Labelbox combines a collaborative interface with powerful machine learning capabilities to ensure efficient labelling processes, supporting text, image, and video data types.
2. Supervisely
This tool specializes particularly in image and video annotation, providing intuitive drawing tools that enhance pixel-perfect labelling and analysis.
3. Amazon SageMaker Ground Truth
Ground Truth is a managed data labeling service that automates aspects of data annotation, which helps expedite the process while also providing human labeling when needed.
4. Snorkel
Snorkel emphasizes programmatic labeling and allows users to define heuristics to label datasets efficiently while also facilitating various annotating strategies.
Best Practices for Successful Data Annotation
While employing a labelling tool, keeping in mind best practices will maximize your return on investment. Here are some guidelines:
1. Create Clear Guidelines
Ensure that all annotators understand the labelling criteria. Having a guide minimizes inconsistencies and maintains standardization across the dataset.
2. Invest in Quality Assurance
Regular checks and feedback loops are crucial. Implement mechanisms to audit and review annotations to uphold quality control.
3. Engage Annotators in the Process
Annotators are crucial to the project; engage them in discussions about challenges and feedback. Their real-world insights can help refine and improve the annotation guidelines.
4. Continually Update Annotation Processes
As your data evolves, so should your annotation processes. Stay updated with the latest trends and technologies in labelling tools to adapt as necessary.
Conclusion: Embracing Labelling Tool Machine Learning
In summary, the implementation of a labelling tool for machine learning is essential for any business looking to harness the power of data-driven decision-making. Through the appropriate choice of a labelling tool, effective practices, and knowledge of your data needs, you can set your business on the path to success. At Keylabs.ai, our dedicated data annotation tools and platforms are designed to support companies like yours in navigating the complexities of data with ease, efficiency, and accuracy.
The future is undoubtedly data-driven, and by utilizing the right labelling tool, your business can thrive in this new landscape. Commitment to quality data annotation can lead to powerful insights, improved models, and, ultimately, a comprehensive understanding of your business environment.
Start your journey into the world of machine learning today, and take advantage of the incredible opportunities that proper data labelling can provide.