Unleashing the Power of **Data Labelling Services**: Your Guide to Data Annotation Excellence
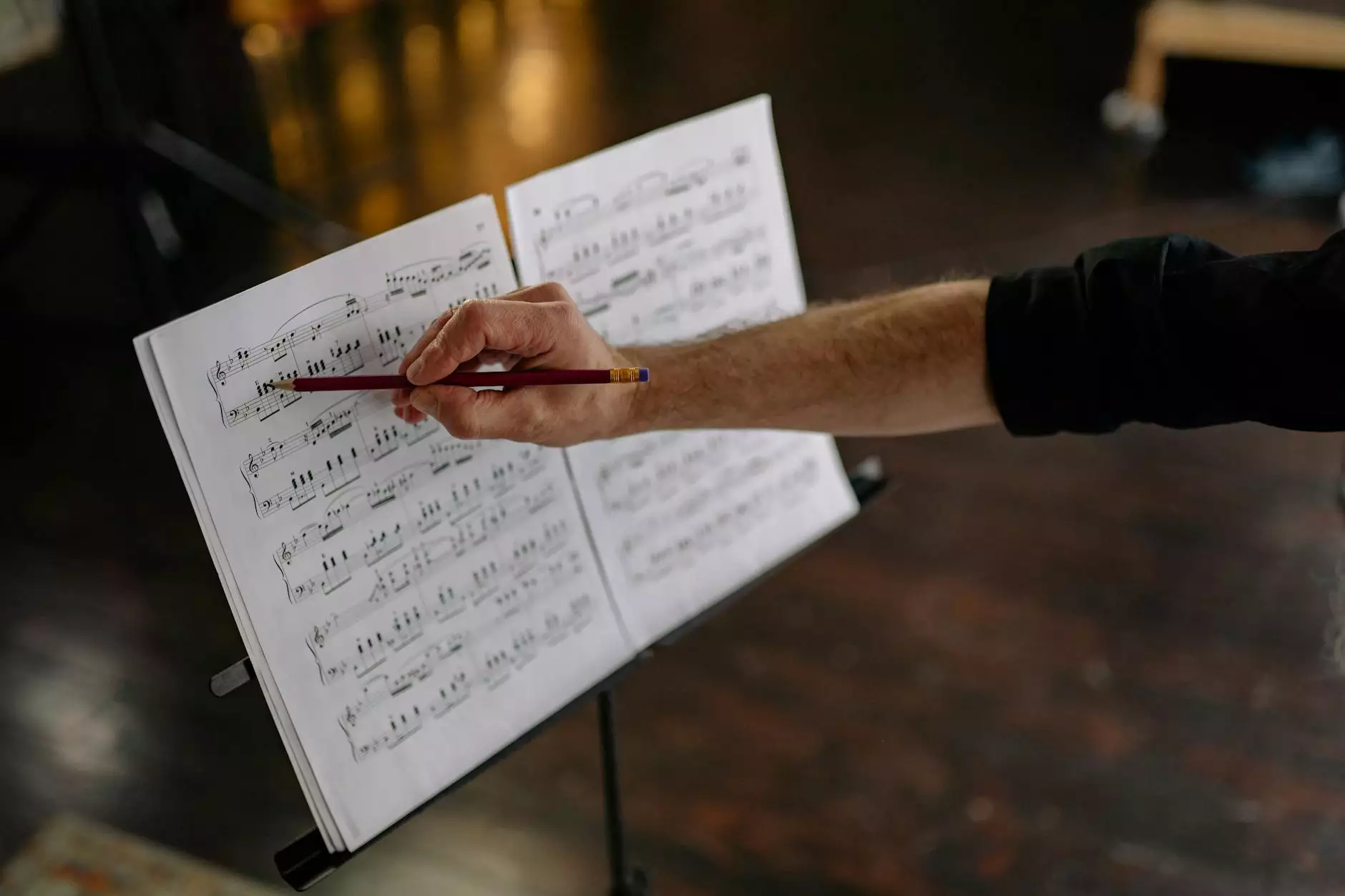
In the age of artificial intelligence and machine learning, the quality of data significantly influences the performance of algorithms. Data labelling services have emerged as a critical component in the data preparation process. As businesses increasingly rely on data-driven decisions, understanding the intricacies of data labelling is essential.
Understanding Data Labelling Services
Data labelling services involve the process of annotating or tagging data to create meaningful labels that can be used for training machine learning models. This ensures that algorithms can learn from high-quality, well-annotated datasets. Without proper labelling, even the most advanced machine learning algorithms can fail to deliver accurate predictions.
The Importance of Data Labelling
The process of data labelling is integral for several reasons:
- Enhances Accuracy: Well-labeled data allows machine learning models to make more accurate predictions and decisions.
- Facilitates Understanding: Annotation helps data science teams understand the context and nuances in data.
- Improves Model Performance: Comprehensive data labelling can significantly enhance model performance, reducing error rates.
- Enables Validation: Labeled data is crucial for validating the output of machine learning models, ensuring they are functioning correctly.
Types of Data Labelling Services
There are various types of data labelling services offered based on the needs of businesses and the nature of the data. Below are the primary types:
1. Image Annotation
Image annotation is the process of labeling images to make them understandable to machine learning models. This can include tasks such as:
- Bounding Boxes: Drawing rectangles around objects of interest within images.
- Segmentation: Dividing an image into segments to identify individual objects.
- Landmarking: Marking specific points of an object, such as facial recognition.
2. Video Annotation
Video annotation involves labeling sequences of images, or video frames, for applications such as surveillance, self-driving cars, and sport analysis. Common techniques include:
- Object Tracking: Labeling objects in motion across multiple frames.
- Action Recognition: Identifying and classifying actions performed in a video.
3. Text Annotation
Text annotation involves tagging and labeling text for natural language processing applications. Key types include:
- Entity Recognition: Identifying and categorizing key elements in the text, such as names, dates, and locations.
- Sentiment Analysis: Labelling text based on emotional tone or sentiment.
- Parts-of-Speech Tagging: Labelling words in context for their grammatical role.
The Role of a Data Annotation Platform
Utilizing a robust data annotation platform is vital for businesses seeking efficient and effective data labelling services. Here’s why a data annotation platform enhances the labelling process:
1. Centralized Management
A data annotation platform consolidates all annotation tasks, making it easier to manage workflows, track progress, and ensure that the annotated data meets quality standards.
2. Collaboration and Scalability
These platforms often provide tools that enable collaboration among team members. This is especially important for large projects that require input from multiple annotators. Additionally, these platforms can scale easily, accommodating growing data needs.
3. Quality Assurance Mechanisms
Effective data annotation platforms include quality assurance processes to review and validate annotated data, minimizing human error and improving the reliability of the dataset.
Best Practices for Using Data Labelling Services
To maximize the benefits of data labelling services, businesses should follow these best practices:
1. Define Clear Objectives
Before initiating the data labelling process, it is crucial to define clear objectives. Understanding the specific goals will help in selecting the right labelling strategy and ensuring that the annotated data aligns with the intended outcomes.
2. Choose the Right Annotation Type
Select the appropriate type of labelling based on the nature of the data and the machine learning model being used. Whether it’s image, video, or text annotation, the choice can have significant implications on the model’s performance.
3. Utilize Skilled Annotators
Providing thorough training to annotators is essential. Skilled annotators who understand the context and nuances of the data can significantly increase the quality of annotations. Additionally, consider leveraging advanced tools that assist annotators in making accurate labels.
4. Implement Quality Controls
Building quality control measures into the annotation process ensures datasets are consistently high quality. This can include random checks, dual annotations, and regular feedback sessions.
Benefits of Partnering with KeyLabs.ai for Data Labelling Services
At KeyLabs.ai, we specialize in providing state-of-the-art data labelling services tailored to meet the unique needs of various industries. Here are just a few of the benefits of partnering with us:
1. Expertise in Various Domains
Our team consists of experts with deep knowledge of diverse fields, enabling us to deliver high-quality annotations for complex datasets across various industries.
2. Advanced Annotation Tools
We utilize cutting-edge data annotation tools that streamline the labelling process and enhance efficiency. Our platforms are designed for user-friendliness and optimized for accuracy.
3. Commitment to Quality
At KeyLabs.ai, quality is our top priority. Our rigorous quality control procedures ensure that every dataset is annotated to the highest standards, ready to fuel your machine learning models effectively.
4. Flexibility and Scalability
Whether you need a small-scale project completed quickly, or you have expansive data sets that require ongoing support, we have the flexibility and resources to meet your needs on time and within budget.
Case Studies: Success Through Effective Data Labelling
Real-world applications of successful data labelling services provide insight into how proper data annotation can revolutionize businesses. Here are a couple of examples:
Case Study 1: Enhancing Autonomous Vehicle Technology
A leading automotive company faced challenges in training their self-driving algorithms due to unannotated video data. By implementing a comprehensive video annotation service through KeyLabs.ai, they managed to:
- Label Over 100,000 Video Clips: Precision tracking and object classification across diverse environments.
- Improve Model Performance by 30%: Enhanced detection capabilities for objects such as pedestrians and traffic signs.
Case Study 2: Revolutionizing E-commerce with AI
An e-commerce platform sought to improve its recommendation algorithms through natural language processing. Partnering with KeyLabs.ai for text annotation, they successfully:
- Annotated Millions of Product Descriptions: Key entities, sentiments, and categories were tagged to train their models.
- Increased Conversion Rates by 25%: Improved relevance of product recommendations led to significantly higher customer engagement.
Conclusion: The Future of Data Labelling Services
As data labelling services continue to evolve, they will play an increasingly critical role in shaping the future of artificial intelligence and machine learning. Companies that invest in high-quality labelling and robust annotation platforms will gain a significant competitive advantage in the data-driven landscape.
By understanding the importance of these services and adopting best practices, businesses can leverage the power of their data, turning it into actionable insights that drive growth and innovation. Explore how KeyLabs.ai can partner with you to enhance your data labelling processes and ensure your machine learning models achieve their full potential.